How to implement predictive analytics in marketing?
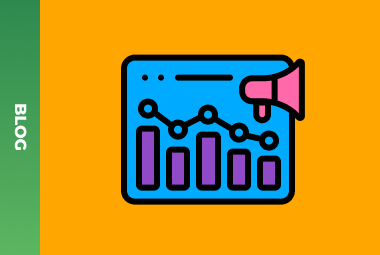
As we draw the curtains on our exploration of data analytics in marketing, one thing becomes abundantly clear: the future of marketing belongs to those who embrace the transformative potential of data-driven strategies.
As marketers (including the author of this article) navigate the ever-shifting currents of the digital age, data analytics stands as a beacon of hope, offering insights, clarity, and direction in an otherwise turbulent sea of information.
In this article, we’ll explore not only the importance of data analytics in marketing but also the key components of predictive analytics that drive success in the ever-evolving marketing landscape. From data collection and integration to predictive modeling marketing, we’ll unravel the intricacies of leveraging data analytics effectively to inform strategic marketing decisions and foster meaningful connections with consumers. Let’s go!
Importance of data analytics in marketing
Data analytics serves as the cornerstone of modern marketing efforts, providing marketers with a deep understanding of their target audience. By analyzing vast volumes of data collected from various sources such as customer interactions, website traffic, and social media engagements, marketers gain actionable insights that fuel strategic initiatives.
So, what exactly does data analytics give marketing? Here’s what:
- Enhanced customer understanding: Marketers gain profound insights into consumer behavior, preferences, and sentiments, allowing for effective audience segmentation and personalized messaging.
- Improved targeting and segmentation: Data analytics enables precise identification and targeting of high-potential audience segments through demographic, psychographic, and behavioral segmentation, maximizing engagement and conversion rates.
- Optimized marketing campaigns: Data-driven insights empower marketers to refine messaging, allocate resources strategically, and optimize campaign performance metrics like click-through rates, conversion rates, and ROI for maximum impact.
- Predictive analytics for future trends: Marketers can forecast future trends and anticipate consumer behavior accurately using predictive models and machine learning algorithms, staying ahead of the competition and identifying emerging opportunities.
- Improved marketing attribution: Accurate attribution of revenue and conversions to specific marketing initiatives is enabled through data analytics, allowing marketers to understand the impact of each touchpoint and allocate resources effectively to maximize ROI.
- Enhanced customer experience: Personalized and relevant experiences across all touchpoints, from recommendations to promotions, enhance customer satisfaction, loyalty, and retention, driven by data-driven marketing initiatives.
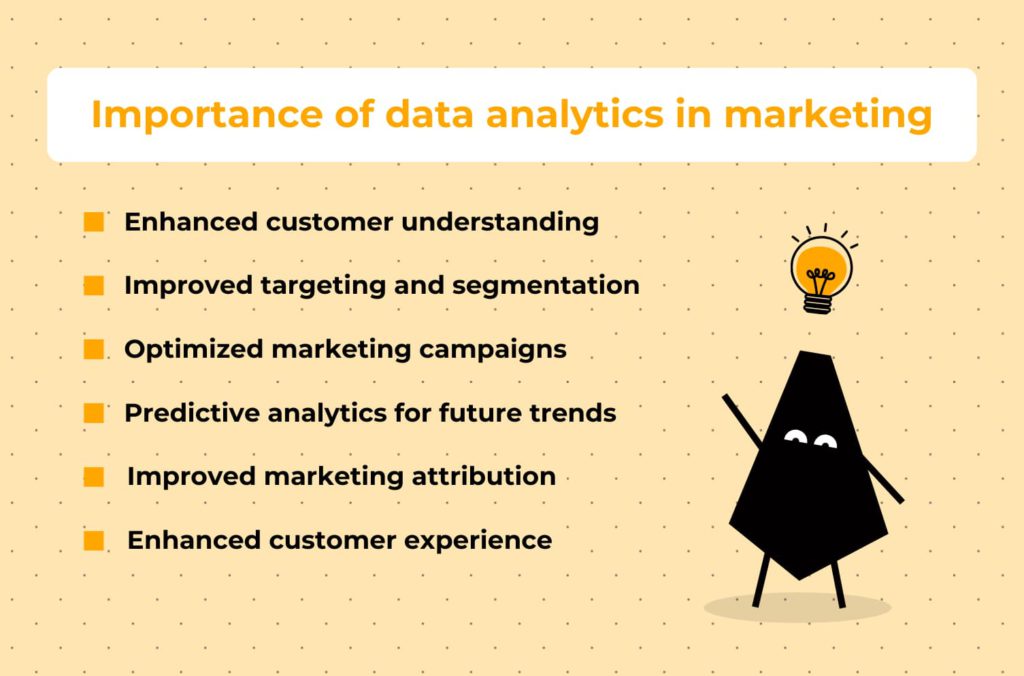
Key components of predictive analytics in marketing
Predictive analytics in marketing encompasses a series of key components that collectively enable marketers to extract actionable insights, forecast trends, and optimize marketing strategies. And understanding these components is essential for harnessing the power of predictive analytics effectively.
Data collection and integration
Data collection and integration serve as the foundational pillars of predictive analytics in marketing. Marketers rely on a diverse array of data sources, including customer databases, website analytics, social media platforms, and third-party data providers, to gather comprehensive insights into consumer behavior and market dynamics.
- Customer databases: Customer databases contain valuable information such as demographic details, purchase history, transactional data, and contact information, providing marketers with deep insights into individual customer profiles and preferences.
- Website analytics: Website analytics platforms track user interactions, website traffic, conversion metrics, and navigation patterns, offering valuable insights into customer engagement, browsing behavior, and conversion funnels.
- Social media platforms: Social media platforms capture user-generated content, sentiment analysis, and social interactions, enabling marketers to gauge brand sentiment, identify influencers, and engage with target audiences in real-time.
- Third-party data providers: External data sources such as market research reports, industry benchmarks, and geographic data enrich marketers’ understanding of market trends, competitive dynamics, and target audience characteristics.
Data cleaning and preparation
Before data can be used for predictive modeling, it must undergo a process of cleaning and preparation to ensure accuracy, consistency, and reliability. Data cleaning involves identifying and correcting errors, inconsistencies, and missing values within the dataset, while data preparation involves transforming and formatting the data for analysis.
- Data cleaning: Data cleaning tasks include removing duplicate records, correcting inaccuracies, filling in missing values, and standardizing data formats to ensure consistency and reliability.
- Data transformation: Data transformation involves converting categorical variables into numerical formats, scaling numerical variables to a common range, and encoding text data for analysis using techniques such as one-hot encoding or label encoding.
- Feature engineering: Feature engineering involves creating new variables or features from existing data that may enhance predictive model performance, such as calculating derived metrics, aggregating data at different levels of granularity, or creating interaction terms between variables.
Predictive modeling techniques
Predictive modeling techniques form the core of predictive analytics in marketing, enabling marketers to build statistical models that forecast future outcomes based on historical data and patterns. Common predictive modeling techniques include:
- Regression analysis: Regression models identify relationships between independent variables and a dependent variable, enabling marketers to predict numerical outcomes such as sales revenue, customer lifetime value, or purchase frequency.
- Classification models: Classification models classify data into distinct categories or classes based on input variables, enabling marketers to predict customer segmentation, churn probability, or response to marketing campaigns.
- Clustering analysis: Clustering algorithms group similar data points together based on shared characteristics, enabling marketers to identify homogeneous customer segments, uncover hidden patterns, and personalize marketing strategies for distinct audience groups.
- Machine learning algorithms: Advanced machine learning algorithms such as random forests, gradient boosting machines, and neural networks learn patterns and relationships from data, enabling marketers to build sophisticated predictive models capable of handling complex datasets and nonlinear relationships.
Navigating the challenges and opportunities of predictive analytics in marketing strategies
Implementing predictive analytics for marketing strategies presents both exciting opportunities and daunting challenges. But fear not, for while the journey may be challenging, the rewards are boundless.
In this section, we’ll be your guides as we navigate through the intricacies of integrating predictive analytics into marketing strategies. We’ll unravel the complexities of selecting the right predictive marketing software, explore the nuances of integrating predictive analytics with existing marketing tools, and uncover the art of building predictive models tailored for targeted campaigns.
Selecting the right predictive marketing software
Navigating the vast ocean of predictive marketing software demands a strategic approach. So, how can you set sail in the right direction?
- Exploring features and capabilities: Take a deep dive into the features and capabilities offered by predictive marketing software. Look for tools that offer advanced data visualization, a range of predictive modeling algorithms, scalability, and intuitive user interfaces.
- Seeking integration compatibility: Navigate towards software solutions that seamlessly integrate with your existing marketing ecosystem. Ensure compatibility with your CRM systems, data management platforms, and other essential tools for efficient data sharing and analysis.
- Charting scalability and flexibility: Plot a course towards software that can scale alongside your organization’s growth. Choose flexible solutions that can adapt to evolving marketing strategies and changing business objectives without compromising performance.
- Anchoring in data security and compliance: Drop anchor with software solutions that prioritize data security and compliance. Choose platforms that adhere to industry standards and regulations to safeguard sensitive customer information and maintain trust.
Integrating predictive analytics with existing marketing tools
Integrating predictive analytics into your existing marketing tools is the bridge between insights and action. Here’s how to make the connection:
- Forging seamless data Iitegration: Forge strong connections between predictive analytics platforms and your existing marketing tools. Establish data pipelines that enable real-time synchronization and analysis for timely insights.
- Navigating workflow automation: Navigate through workflow automation tools to streamline data processing, model deployment, and campaign execution. Automate repetitive tasks to accelerate time-to-insight and free up resources for strategic initiatives.
- Extending insights across channels: Extend the reach of predictive analytics across all marketing channels. From email campaigns to social media ads, leverage insights to deliver personalized experiences and maximize engagement.
- Monitoring performance and optimization: Keep a vigilant eye on the performance of your predictive models. Monitor campaign results and optimize strategies based on actionable insights to drive continuous improvement and maximize ROI.
Need help implementing predictive analytics into your software?
Building predictive models for targeted campaigns
Crafting predictive models for targeted campaigns is the compass that guides you from insight to action. Here’s your roadmap:
- Exploring data insights: Embark on a journey of exploration through your data. Identify key variables and insights that will drive the success of your targeted campaigns.
- Selecting the right modeling techniques: Choose the modeling techniques that best align with your campaign objectives and audience characteristics. Select tools that empower you to turn data into actionable insights.
- Validating and refining models: Validate your models using rigorous metrics and techniques. Refine your models based on real-world feedback and insights to ensure accuracy and reliability in predictive outcomes.
- Implementing predictive strategies: Implement your predictive models into your marketing campaigns and decision-making processes. Seamlessly integrate them into your workflows to maximize impact and drive meaningful results.
The role of Adtech software in predictive marketing
Adtech software plays a pivotal role in the realm of predictive marketing, facilitating targeted advertising campaigns, optimizing ad placements, and maximizing return on investment (ROI). Here’s a closer look at its significance.
Understanding audience segmentation
Adtech software enables marketers to segment their target audience based on various demographics, behaviors, and interests. By leveraging predictive analytics, adtech platforms can identify patterns and trends within audience data, allowing marketers to create highly targeted and personalized advertising campaigns.
Real-time bidding and ad placement
With real-time bidding (RTB) capabilities, adtech software allows marketers to bid on ad inventory in real-time auctions. Predictive algorithms analyze vast amounts of data, including user behavior and ad performance metrics, to determine the optimal bid for each impression. This dynamic bidding process ensures that ads are placed in front of the right audience at the right time, maximizing engagement and conversion rates.
Predictive campaign optimization
Adtech software employs predictive modeling techniques to optimize advertising campaigns continuously. By analyzing historical campaign data and performance metrics, predictive algorithms can identify trends, patterns, and insights to improve targeting, messaging, and creative elements. This iterative optimization process helps marketers enhance campaign effectiveness and achieve their marketing objectives.
Cross-channel advertising integration
In today’s multi-channel digital landscape, adtech software enables seamless integration across various advertising channels, including display ads, social media advertising, search engine marketing (SEM), and video advertising. Predictive analytics allows marketers to orchestrate cohesive cross-channel advertising campaigns, ensuring consistent messaging and maximizing reach and impact across all touchpoints.
Attribution modelling and performance measurement
Adtech software provides robust attribution modeling capabilities, allowing marketers to accurately measure the impact of their advertising efforts across the customer journey. Predictive attribution models analyze data from multiple touchpoints and channels to attribute conversions and sales accurately. This insight enables marketers to allocate budget effectively, optimize campaign performance, and maximize ROI.
Revolutionize your adtech strategies now! Explore the power of data-driven insights for unparalleled advertising success
Conclusion
Throughout our journey, we’ve uncovered the myriad ways in which data analytics empowers marketers to decode consumer behavior, predict trends, and optimize campaigns with precision and insight. But our exploration doesn’t end here. As we stand at the precipice of a data-driven future, the opportunities for innovation and growth are boundless. By harnessing the power of data analytics, marketers can navigate the complexities of an ever-evolving landscape, adapt to changing consumer preferences, and stay ahead of the curve in a competitive market.
So let us forge ahead with confidence, knowing that the future of marketing is ours to shape, and the journey ahead is filled with infinite opportunities for growth, success, and impact.