The risks of AI adoption in healthcare and how to address them
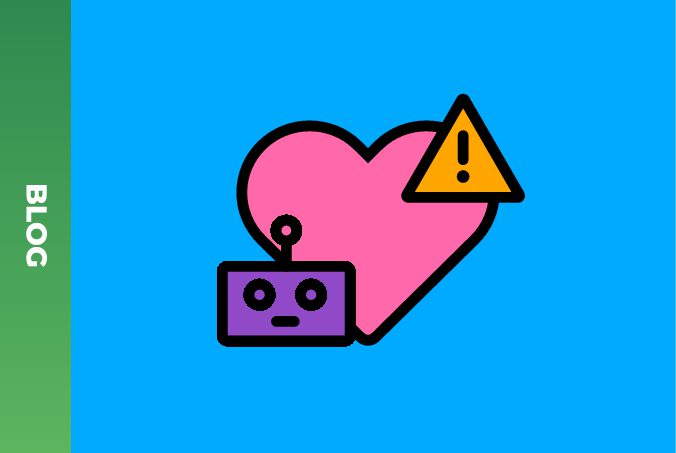
Would an electric light bulb have been invented if Edison had not conducted thousands of experiments or faced numerous failures? His risk-taking and perseverance revolutionized the way our world is illuminated. Similarly, in the same vein, the story of Steve Jobs’s ability to take calculated risks in his efforts for Apple can be added. The same situation is with Artificial Intelligence (AI) adoption in healthcare. Whether you apply AI or BI, there are risks of it, of course. Our job is to take, manage, mitigate reasonably, and turn into our benefit.
Some interesting figures: As per Statista, according to a survey of healthcare provider executives in the United States in 2023, almost 30 percent cited adopting artificial intelligence for clinical decision support tools as a priority. Furthermore, a quarter said an AI use case for predictive analytics and risk stratification was a priority. According to another source, around 1/5 of healthcare organizations have already adopted AI models for their healthcare solutions.
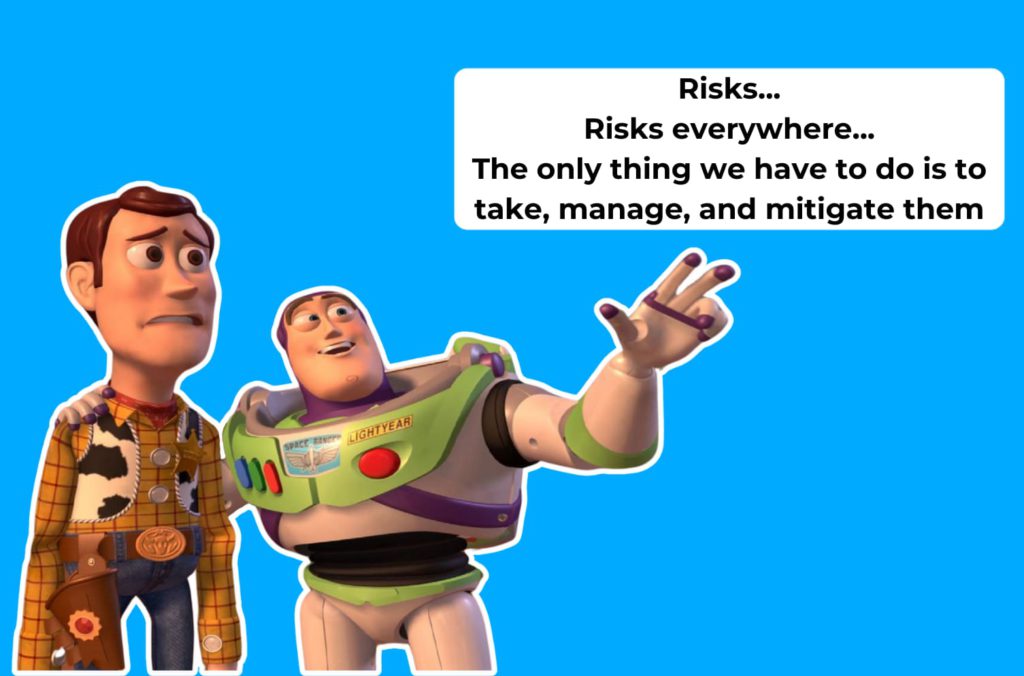
This AI adoption trend is expanding like the Universe. The reason healthcare providers adopt AI in healthcare is because of its possibilities, promising improved diagnostics, streamlined processes, and enhanced patient care. However, AI cuts both ways. For instance, some patients are uncomfortable with their provider relying on AI. Why is it so? In this article, we identify the risks of AI adoption, consider the pros and cons of AI adoption in healthcare, explore the potential pitfalls, and propose strategies to address them.
Pros and cons of AI in healthcare
Let’s start with the advantages of this journey. The integration of AI in healthcare brings about numerous positive aspects, revolutionizing the way medical professionals deliver care and improving patient outcomes. Here are some pros of AI in healthcare:
Personalized care and treatment plans
Generative AI contributes to personalized healthcare by its ability to analyze and process individual patient data allowing for the development of personalized treatment plans based on the patient’s unique medical history, genetic makeup, and lifestyle factors. Personalization improves treatment effectiveness and minimizes adverse effects, contributing to better patient outcomes.
Natural Language Processing (NLP) for data analysis
NLP enables the extraction of meaningful insights from unstructured medical data, such as clinical notes and research papers. Analyzing unstructured data enhances the depth of information available to healthcare professionals, aiding in research and clinical decision-making.
Precision diagnostics
AI algorithms can analyze vast datasets, including medical records, imaging studies, and genomic information, to provide more accurate and timely diagnoses. By identifying patterns and anomalies, AI enhances the precision of diagnostics, leading to earlier detection of diseases and improved treatment planning.
Operational efficiency
Automation of routine administrative tasks, such as appointment scheduling, billing, and data entry, frees healthcare professionals to focus on patient care. Improved operational efficiency leads to cost savings, optimized resource allocation, and enhanced overall healthcare management.
Predictive analytics and preventive care
AI can analyze patient data to identify patterns and trends, enabling the prediction of potential health risks. Predictive analytics aids in preventive care by allowing healthcare providers to intervene early, reducing the likelihood of complications and improving long-term patient health.
Remote patient monitoring
AI-powered devices enable continuous remote monitoring of patients, allowing healthcare providers to track vital signs and receive real-time data. Remote monitoring enhances patient engagement, facilitates early intervention, and is particularly beneficial for those with chronic conditions.
Virtual health assistants
AI-driven virtual assistants and chatbots provide patients with instant access to healthcare information, appointment scheduling, and medication reminders. These technologies improve patient engagement, accessibility, and adherence to treatment plans.
Image and speech recognition
AI technologies, particularly in image and speech recognition, assist in the interpretation of medical images, such as X-rays and MRIs, and transcription of clinical notes. Faster and more accurate analysis of medical images enhances diagnostic capabilities and reduces the time spent on administrative tasks.
Drug discovery and development
AI accelerates the drug discovery process by analyzing biological data, identifying potential drug candidates, and predicting their efficacy. Streamlining drug development leads to faster introduction of new treatments, potentially addressing unmet medical needs and improving patient care.
Enhanced surgical assistance
AI assists surgeons by providing real-time information during procedures, aiding in precision and minimizing errors. Surgical robots, guided by AI, can perform complex procedures with greater accuracy, reducing recovery times and improving patient outcomes.
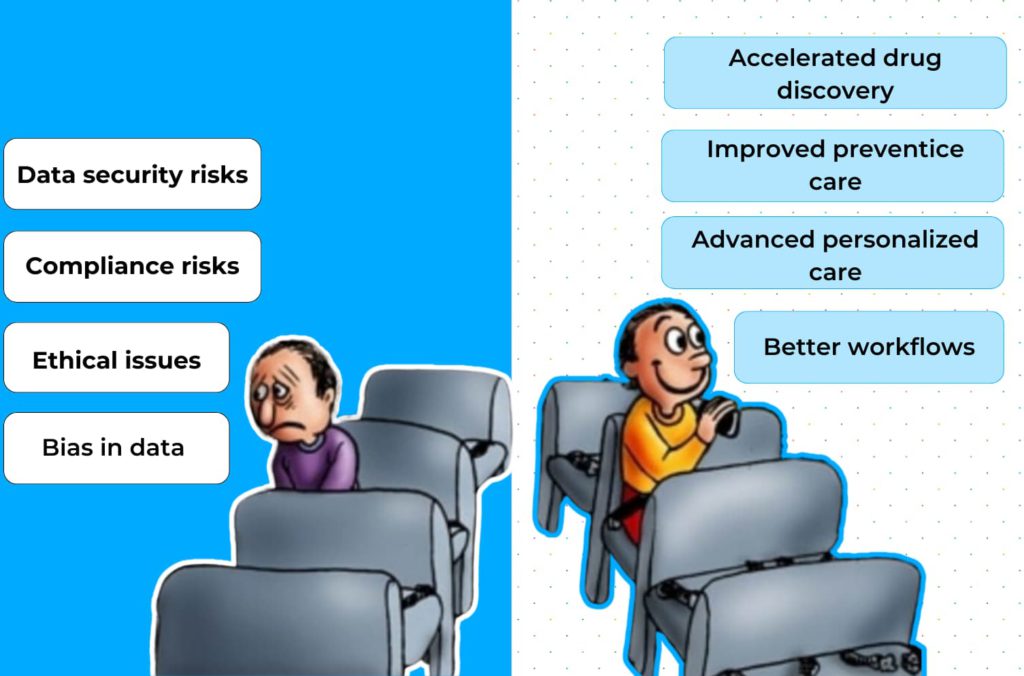
While AI shows its bright side in healthcare, it is important to acknowledge its dark side too, identify and address the potential drawbacks and challenges associated with its implementation. Let’s face them:
Data privacy and security
No doubt AI influences healthcare data security. The generation of synthetic data raises concerns about privacy and security, as the generated content may inadvertently reveal sensitive information. AI systems require access to large amounts of sensitive patient data. The risk of data breaches or unauthorized access increases, posing a threat to patient privacy.
Addressing data security and privacy risk of AI in healthcare: Implementing robust data security measures is imperative to safeguard patient information. Encryption protocols, secure data storage, and access controls must be implemented to prevent unauthorized access. Additionally, healthcare organizations need to prioritize continuous monitoring and periodic security audits to identify and address potential vulnerabilities promptly.
Regulatory compliance
The use of generative AI in healthcare must comply with existing regulatory frameworks, such as the Health Insurance Portability and Accountability Act (HIPAA) in the United States. Ensuring compliance is critical to maintaining the confidentiality and integrity of patient information.
Addressing regulatory compliance risk of AI in healthcare: Navigating the complex landscape of healthcare regulations requires a meticulous approach. Healthcare organizations must stay informed about evolving regulations, update their practices accordingly, and integrate robust compliance programs. Also, it is required to establish standards that encompass ethical considerations, data sharing protocols, and transparency requirements. Collaboration with regulatory bodies and legal experts is essential to navigate the intricate legal frameworks surrounding AI in healthcare.
Bias in data
In healthcare, biased algorithms can lead to disparities in patient outcomes, diagnosis, and treatment recommendations. For instance, if historical data used to train an AI model is skewed towards a particular demographic, the algorithm may not perform effectively for other groups.
Addressing bias in data risk of AI in healthcare: Mitigating bias requires a multifaceted approach. First, diverse and representative datasets should be used to train AI algorithms, ensuring that they encompass a broad spectrum of demographic and clinical information. Data shall be prepared for AI thoroughly. Regular audits of AI algorithms must be conducted to identify and rectify any bias that may emerge over time. Moreover, involving multidisciplinary teams, including ethicists and social scientists, in the development process can provide a more comprehensive perspective on potential biases.
Learning from AI failures in healthcare
AI failures, whether in diagnostic accuracy or treatment recommendations, underscore the need for continuous monitoring and improvement. Collaborative efforts between healthcare professionals and AI developers are essential to refine algorithms and enhance their reliability.
Understanding AI adverse events in healthcare
In the face of AI failures and adverse events, organizations must be proactive in implementing corrective measures. This involves acknowledging shortcomings, learning from mistakes, and transparently communicating the steps taken to rectify issues. These issues also include ethical ones.
Ethical issues with AI in healthcare
Discriminatory hiring algorithms
- Issue: AI-powered hiring algorithms may inadvertently perpetuate gender and racial biases present in historical hiring data.
- Unethical AI example: Amazon faced scrutiny when it was revealed that its recruiting AI system was biased against women. The system had been trained on resumes submitted over a ten-year period, which predominantly came from male applicants.
Facial recognition biases
- Issue: Facial recognition algorithms have demonstrated biases, especially against people of color. These biases can lead to discriminatory outcomes and reinforce existing prejudices.
- Unethical AI example: In 2018, it was reported that some facial recognition systems exhibited gender and racial biases, resulting in misidentification and discriminatory actions against certain demographic groups.
Autonomous vehicles and ethical dilemmas
- Issue: Autonomous vehicles must make split-second decisions in ethically complex situations, leading to concerns about how AI algorithms prioritize different lives or scenarios.
- Unethical AI example: The ethical considerations of self-driving cars have been widely discussed. For instance, the “trolley problem” scenarios raise questions about how autonomous vehicles should prioritize the safety of occupants versus pedestrians in emergencies.
Conclusion: the risks and disadvantages of AI in healthcare can be covered by mitigation strategies
The integration of AI in healthcare is a double-edged sword and unavoidable, offering immense potential for innovation while posing significant risks. To navigate this complex landscape successfully, healthcare organizations must prioritize ethical considerations, address biases, implement stringent data security measures, and learn from AI failures. Balancing innovation with patient safety requires collaborative efforts between regulatory bodies, healthcare professionals, and technology developers.
As the healthcare industry keeps evolving, a proactive and responsible approach to AI adoption is crucial. By addressing the risks head-on and implementing effective mitigation strategies, the healthcare sector can harness the transformative power of AI while upholding the highest standards of patient care and ethical practice. Are any concerns left behind? Just contact us for relief. See you soon!