How to Get Data Ready for Artificial Intelligence in Healthcare
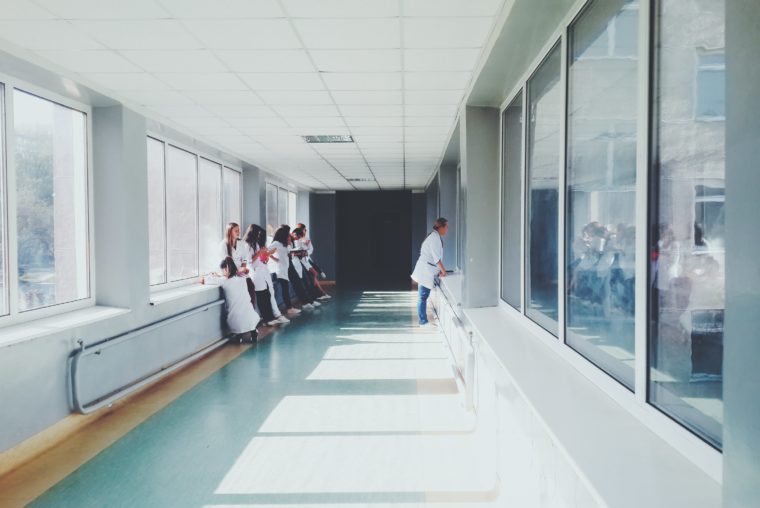
Healthcare industry is eager to implement artificial intelligence (AI), machine learning (ML), natural language processing (NLP) and other so-called ‘big data’ related tools and solutions. This is understandable as, in healthcare the potential value that could be unlocked from data is enormous.
In this article, we look at the role of AI in healthcare, and why data readiness and preparation is essential for making AI projects possible. Accenture research estimates that globally AI can grow economic output by $14 trillion, with a possible impact on corporate profitability, up 38% by 2035.
In healthcare, AI is already being used to improve and enhance diagnostic techniques, process patient data more effectively, and reduce the impact of human error across administrative functions. But these are only a few ways it could be used, and data itself is the key to making future leaps forward possible. In another Accenture survey, “18 percent of health executives say that one of the main obstacles to broad-scale AI implementation”, is data readiness.
Future of AI in Healthcare
Humanity, at our current level of technological development, is on the way to developing true AI. We are not there yet. True AI is when a machine can learn for itself, entirely independent of human involvement and input. At present in the broad context, AI is interchangeable with machine learning (ML); however, there is a distinction which is useful to note. AI is a multi-disciplinary attempt to create self-teaching machines.
Whereas, ML is a machine/software that can learn from experience, with algorithms at the heart of a system that is constantly learning. Both are heavily reliant on data; so with larger, cleaner data-sets, these evolving systems can learn faster, evolve, and start unlocking real value from the data being absorbed. When it comes to the role of AI in healthcare, this relates to the way algorithms, mathematical modeling, and data clustering can be used to extract significant, meaningful value from large data sets.
Why is preparation key for AI readiness?
AI and ML can be implemented in the healthcare sector and any industry which uses and processes large amounts of data. One of the main challenges is that when companies want to go ahead with these sorts of projects, the data often lets them down.
Organizational leaders are usually keen to implement AI/ML projects. Upsides of being successful include new operational efficiencies, the ability to create new products or services using data, and systems being able to gain insights and understandings that humans can’t.
Prescriptive analytics is one such example of AI in healthcare. Prescriptive analytics is a phrase coined when IBM launched the ILOG CPLEX Optimization Studio in 2010, before Watson and other AI-powered systems were launched.
AI and ML have advanced considerably since 2010, and one area where they have is using innovations in these fields to make positive impacts in the healthcare sector. Results have demonstrated that hospital readmissions within 30 days can be reduced, illnesses can be diagnosed more accurately, and risks assessed more effectively. One University of Pennsylvania study found that “machine learning and EHR data helped to identify patients on track for severe sepsis or septic shock 12 hours before the onset of the condition.”
Other ways predictive analytics and ML tools are used are to predict when patients aren’t going to show up. No-shows cost healthcare organizations money, and waste valuable time. A Duke University study uncovered “an additional 4800 patient no-shows per year with higher accuracy than previous attempts to forecast patient patterns.”
Before new AI projects are launched, the main challenges that healthcare organizations run up against, is the state of data readiness before they can start. Most healthcare organizations aren’t ready when they start, which means it can take significantly longer to achieve results that organizational leaders expect.
How to prepare data for AI in healthcare?
Business leaders and decision-makers in healthcare are often quite clear on what is needed when it comes to data-related projects. Many know that a data lake, warehouse, or another form of storage is needed to make the raw data useful across an Enterprise organization. This way, the data from one or more departments could prove useful in multiple ways.
Healthcare organizations need to take the following steps to make sure data can be used in AI-based systems:
#1: Clearly define the goals of any AI projects, the desired goals and objectives.
#2: Understand what the data contains, where it currently lives, what and how it needs to be processed and cleaned in order to help achieve the project outcomes and objectives.
#3: Find the right partners to prepare data. To make data easy-to-use, it needs to be cleaned, before it can go into a warehouse and then filter into the application layer, or any other tech stack. This is time-consuming work. Rather than shy away from it, healthcare organizations need to know it takes the work of data scientists and others with a keen understanding of the unique data challenges healthcare organizations face.
Preparing the data is a mission-critical step. How this is done depends on the data itself, and the overall end-goals of the project. It could involve a range of skills and services, such as data architecture, data engineering, and ongoing analytics and insights. Also, for the healthcare sector, this data needs to be processed in such a way as to ensure its PHI and HIIPA compliant.
In this case, here at GreenM, we have worked with partners and health IT companies to prepare and process patient feedback for services delivered, and then that was filtered back to stakeholders and care providers. For another client, real-time alerts were established to warn providers of negative experiences (and positive ones), to ensure that care provisions were always meeting the standards patients expected.
Key takeaways
Regardless of the sophisticated tools and systems brought in to extract actionable insights, the data needs to be made ready first. Firm foundations need to be established. Once data is cleaned and ready, then progress can be made using new solutions such as AI, ML, NLP, and other technology to extract actionable and useful insights from healthcare data.
LEARN HOW TO CREATE ADVANCED BUSINESS INTELLIGENCE SYSTEM
How to get system logs from all clients and build comprehensive analytics to track usage, device failure and performance.