How to effectively use data analytics in the automotive industry?
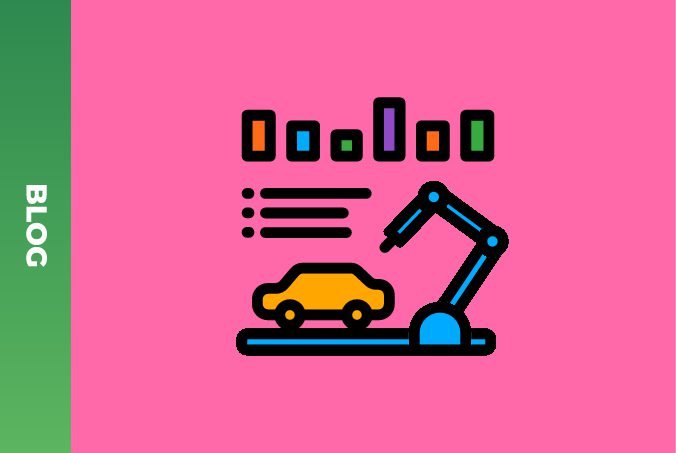
To drive the automotive industry maneuvering, analyze the road ahead. Data analytics holds immense promise for optimizing a supply chain with data visualization, forecasting demand with predictive analytics, and ultimately refining raw data into dollars with actionable insights.
Some interesting facts: The big data market in the automotive industry is expected to grow from USD 5.92 billion in 2024 to USD 12.86 billion by 2029, at a CAGR of 16.78%. The ability to harness data is enabling masses of data to be utilized to form actionable customer segments and individualized offers and incentives to boost sales and improve customer retention. The successful adoption of big data analytics, however, poses several barriers for original equipment manufacturers (OEMs). Particularly, OEMs acknowledge that data is a potentially new source of revenue.
By unlocking the power of big data and advanced analytics techniques, automotive companies can improve their pricing strategies, predictive maintenance, vehicle performance, and emerging technologies. This article is about using data analytics efficiently to drive innovation, enhance operational productivity, and gain a competitive edge.
Wanna rev up your automotive strategy with data analytics?
Why does automotive data analytics matter?
Data means more than you might think. It hides patterns, trends, and preferences from manufacturing and supply chain management to sales, marketing, and customer service. Data doesn’t speak for itself. It should be analyzed, processed, and translated into meaningful actions. You can uncover all of this.
What automotive big data can be brought to analysis?
Let’s see how much data you might skip and leave behind while it could be transformed into valuable things.
Manufacturing data: Data generated during the manufacturing process, including production rates, quality control metrics, equipment performance, and supply chain logistics, can be analyzed to optimize manufacturing efficiency and quality.
Service and maintenance data: Data on vehicle maintenance schedules, service history, warranty claims, and repair costs can be analyzed to optimize preventive maintenance programs, reduce downtime, and improve overall vehicle reliability and customer satisfaction.
Connected car data: Data generated by connected car technologies, such as GPS navigation, infotainment systems, and vehicle-to-vehicle communication, can be analyzed to improve driver experiences, enhance safety features, and enable new services and applications.
Sales and marketing data: Data on sales trends, market demand, advertising effectiveness, and customer interactions can be analyzed to optimize pricing strategies, target marketing campaigns, and identify opportunities for revenue growth.
Supply chain data: Data related to the supply chain, including inventory levels, supplier performance, transportation logistics, and demand forecasting, can be analyzed to optimize inventory management, reduce costs, and improve delivery times.
Vehicle telematics data: Data collected from sensors embedded in vehicles can provide insights into vehicle performance, usage patterns, fuel efficiency, and maintenance needs.
Customer data: Information about customers, including demographics, purchase history, preferences, and feedback, can be analyzed to personalize marketing efforts, improve sales strategies, and enhance customer satisfaction and retention.
Financial data: Financial data, including revenue, expenses, profitability, and return on investment (ROI), can be analyzed to assess business performance, identify cost-saving opportunities, and make informed investment decisions.
Safety and compliance data: Data related to vehicle safety standards, regulatory compliance, recalls, and accident reports can be analyzed to ensure compliance with regulations, identify potential safety issues, and improve overall vehicle safety.
Environmental data: Data on emissions levels, energy consumption, and environmental impact can be analyzed to develop more sustainable automotive technologies, reduce environmental footprints, and meet regulatory requirements.
What are automotive analytics solutions?
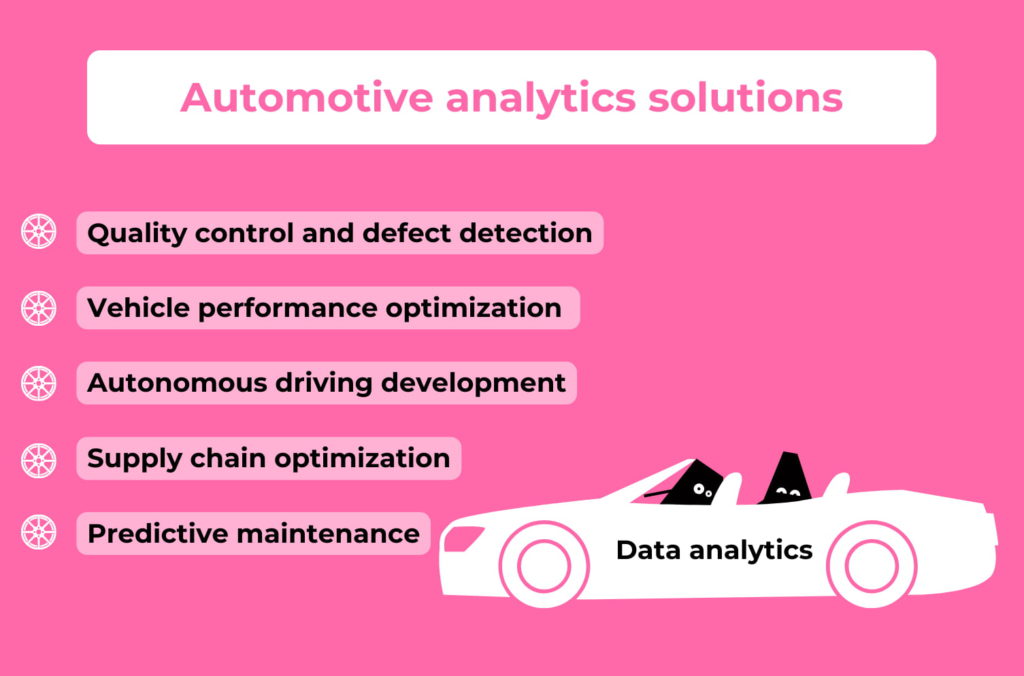
Automotive analytics solutions encompass a range of technologies and methodologies designed to analyze and derive insights from big data of the automotive industry. These solutions leverage advanced analytics techniques, machine learning algorithms, and data visualization tools to extract actionable insights from various data sources. Here are some of them:
Predictive maintenance: By analyzing vehicle sensor data, historical maintenance records, and vehicle diagnostics, automotive companies can predict when components are likely to fail and schedule maintenance proactively, reducing downtime and minimizing repair costs.
Supply chain optimization: Data analytics can optimize supply chain processes by identifying inefficiencies, reducing lead times, optimizing inventory levels, and improving supplier performance, ultimately enhancing production efficiency and reducing costs.
Vehicle performance optimization: Analyzing data from onboard sensors and telematics systems and other relevant data sources allows manufacturers to optimize vehicle performance, improve fuel efficiency, enhance safety features, and diagnose issues remotely, leading to better overall vehicle quality and customer satisfaction.
Quality control and defect detection: By analyzing data from manufacturing processes, quality inspections, and customer feedback, defects can be identified early in the production process, corrective actions can be implemented, and overall product quality can be improved, reducing recalls and warranty costs.
Autonomous driving development: Data analytics plays a crucial role in developing and refining autonomous driving technologies by analyzing vast amounts of data collected from sensors, cameras, and other sources to improve decision-making algorithms, enhance safety features, and optimize vehicle performance in real-world conditions.
Customer Relationship Management (CRM): CRM analytics solutions analyze customer data, including demographics, purchase history, preferences, and behavior, to segment customers, personalize marketing efforts, and improve customer engagement and retention.
Why do you need analytics in the automotive industry?
Customer insights and personalization: Data analytics enables automotive companies to analyze customer preferences, behavior, and feedback to personalize marketing efforts, develop targeted advertising campaigns, and offer customized products and services tailored to individual needs and preferences.
Risk management and fraud detection: Data analytics can help automotive companies identify and mitigate risks related to fraud, warranty claims, supply chain disruptions, and cybersecurity threats, safeguarding against potential financial losses and reputational damage.
Sales and marketing optimization: By analyzing sales data, market trends, advertising effectiveness, and customer interactions, automotive companies can optimize pricing strategies, target the right audience segments, and allocate marketing resources more effectively to drive sales and identify opportunities for revenue growth.
Regulatory compliance: This includes analyzing data related to vehicle safety standards, emissions regulations, recalls, and accident reports to ensure compliance with regulations, identify potential risks, and improve overall safety and regulatory compliance.
Financial and operational analytics: Financial and operational analytics is about analyzing financial data, operational metrics, and key performance indicators (KPIs) to assess business performance, identify cost-saving opportunities, and make informed investment decisions.
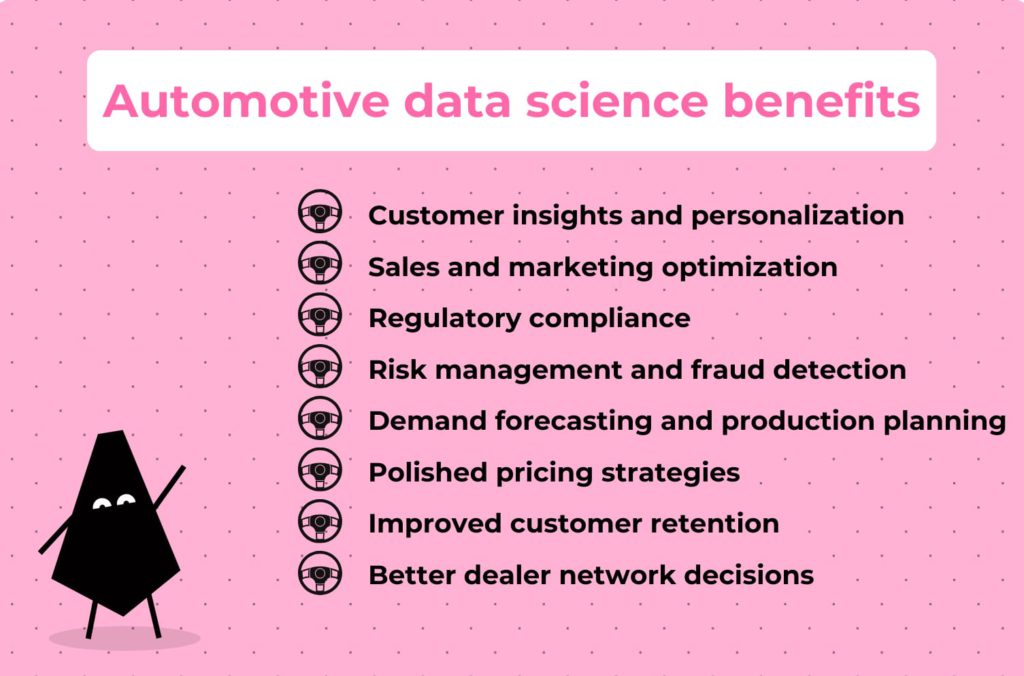
More benefits of data science in the automotive industry
- Demand forecasting and production planning:
- Data sources: Historical sales data, market research data, economic indicators, customer surveys, and other relevant datasets.
- Methodology: Statistical modeling techniques such as time series analysis, regression analysis, and machine learning algorithms are used to analyze historical sales data and identify patterns and trends. Market research and economic data help to understand external factors influencing demand. These insights are then used to forecast future demand for different vehicle models and variants.
- Tools: AI-powered predictive analytics tools, specialized demand forecasting software, and enterprise resource planning (ERP) systems with forecasting modules.
- Polished pricing strategies:
- Data sources: Market pricing data, competitor pricing data, historical sales data, customer segmentation data, and consumer behavior data.
- Methodology: Data analytics techniques such as price elasticity analysis, competitive benchmarking, and customer segmentation are used to understand market dynamics, competitor pricing strategies, and customer preferences. This information is then used to develop pricing strategies that maximize profitability while remaining competitive in the market.
- Tools: Pricing optimization software, data visualization tools, CRM systems, and business intelligence platforms.
- Improved customer retention:
- Data sources: Customer transaction data, CRM data, customer feedback data, social media data, and demographic data.
- Methodology: Data analytics techniques such as customer segmentation, churn analysis, sentiment analysis, and predictive modeling are used to understand customer behavior, preferences, and satisfaction levels. By analyzing this data, automotive companies can identify opportunities to improve customer experiences, personalize marketing efforts, and implement targeted retention strategies.
- Tools: CRM systems, customer analytics software, sentiment analysis tools, marketing automation platforms, and customer feedback management tools.
- Better dealer network decisions:
- Data sources: Dealer performance data, sales data, market demand data, geographic data, and competitor data.
- Methodology: Geographic information system (GIS) analysis, market penetration analysis, and dealer performance benchmarking are used to assess the performance of existing dealerships and identify potential areas for expansion or optimization. Market demand data and competitor analysis help to understand market dynamics and competitive positioning.
- Tools: GIS software, AI solutions, business intelligence tools, market research platforms, and dealership management systems.
Conclusion: big data analytics in the automotive industry is a must
So, the merit of turning raw data into actionable insights and, ultimately, into revenue stands with big data analytics, whether it comes to data analytics for car dealers, car manufacturers, or other stakeholders. Embracing data analytics is essential for navigating the complexities of the automotive industry and achieving long-term success.