What is predictive analytics in healthcare, and its efficient usage
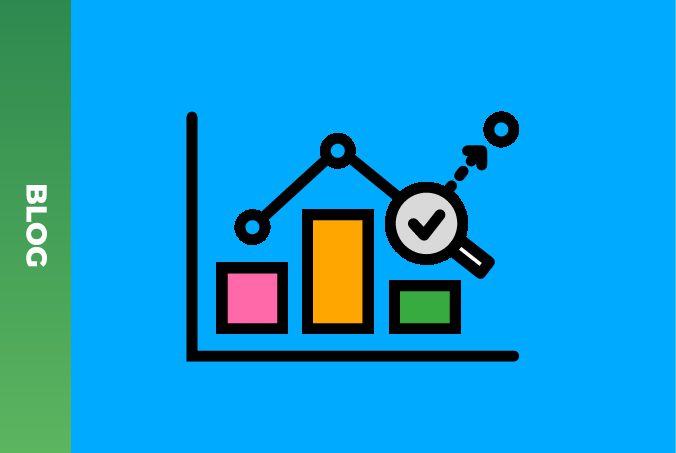
Everyone loves to have things under control. In healthcare, healthcare providers take care of patients by not merely having control over but even predicting patient outcomes. Imagine a healthcare system that not only reacts to medical issues but anticipates and mitigates them before they escalate. Predictive analytics brings this vision closer to reality by leveraging data-driven insights to forecast outcomes, identify risk factors, and streamline healthcare processes.
Some interesting figures: According to Statista’s survey, as of February 2022, Singapore leads in healthcare predictive analytics adoption rate at 92%, followed by China at 79%, and Brazil and the United States at 66%. As per Fortune Business Insights, the global healthcare predictive analytics market size is projected to grow from $10.06 billion in 2022 to $69.63 billion by 2029, at a CAGR of 31.8%.
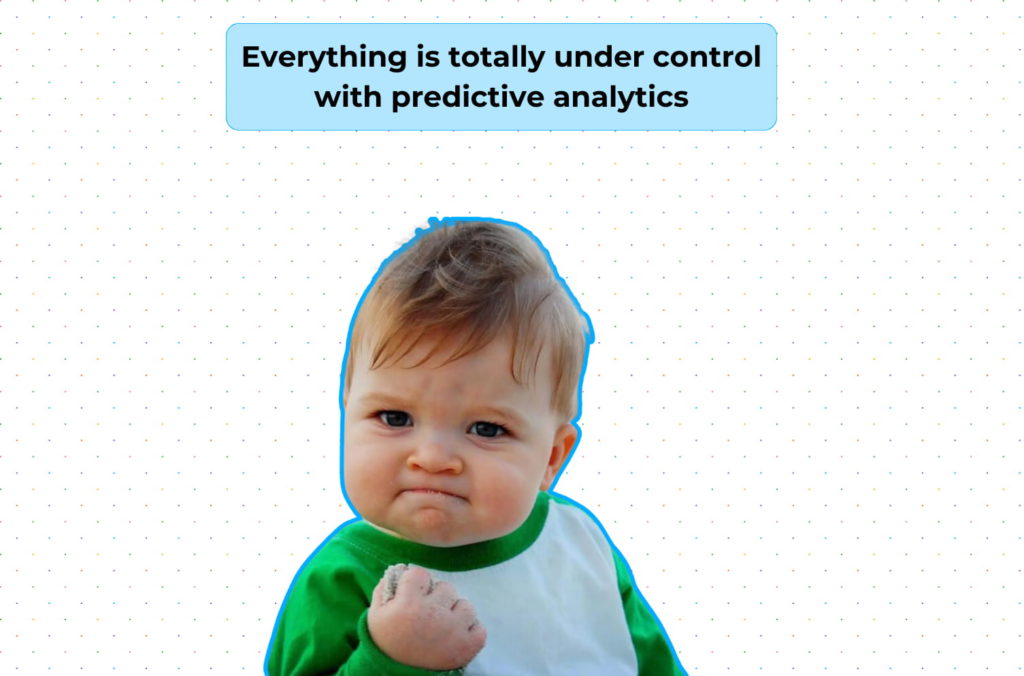
These numbers show that predictive analytics kicks into high gear. From early disease detection to personalized treatment plans and optimized resource allocation, predictive analytics empowers healthcare providers to proactively address challenges. From this article, you will learn what predictive analytics in healthcare is, its inners, and how it works.
Take control of healthcare outcomes with predictive analytics for proactive patient care
Meaning and place of predictive analytics in healthcare
Predictive analytics is revolutionizing the landscape of healthcare by providing a proactive approach to patient care. In essence, it involves the use of statistical algorithms and machine learning models to analyze historical data and identify patterns, trends, and correlations. In healthcare, this means leveraging data from electronic health records (EHRs), medical imaging, patient demographics, and other sources to make predictions about patient health, disease risk, or treatment outcomes.
The primary goal of predictive analytics in healthcare is to assist healthcare professionals in making informed decisions by providing insights into potential future events. However, in some other cases, it can even facilitate turning raw data into revenue. By identifying patterns and trends in data, predictive analytics enables proactive intervention, personalized treatment plans, and resource optimization. So, let’s see how it works in practice.
Applications of predictive analytics in healthcare
Hospital resource management
Healthcare facilities can use predictive analytics to forecast patient admissions, allocate resources efficiently, and streamline operations. By anticipating patient needs, hospitals can optimize staffing levels, reduce wait times, and ensure that resources are allocated where they are most needed. Also, forecasting patient volumes and predicting wait times in emergency departments to optimize resource allocation and improve patient flow.
One more thing here is predicting the demand for medical supplies, equipment, and staffing to optimize resource allocation and avoid shortages.
Medical decision making
Integrating predictive models into clinical workflows assists healthcare professionals in making informed decisions about patient care. This leads to personalized care, i.e. by analyzing patient data, including genetic information, treatment responses, and lifestyle factors, predictive analytics helps in tailoring treatment plans to individual patients. This personalized approach can optimize the effectiveness of treatments, minimize side effects, and enhance overall patient satisfaction.
Patient readmission prediction
Speaking of patients further, predictive analytics models predict the likelihood of a patient being readmitted to the hospital after discharge. This information allows healthcare providers to implement targeted interventions, such as post-discharge monitoring or follow-up care, to reduce the risk of readmission.
Early disease detection
Predictive analytics is used to identify individuals at risk of developing specific diseases based on their medical history, genetic predispositions, and lifestyle factors. Early detection allows for timely intervention and preventive measures, potentially reducing the severity of the disease and improving patient outcomes.
Fraud detection and billing optimization
Predictive analytics plays an important role in identifying potential fraudulent activities, such as billing irregularities or insurance fraud. By analyzing patterns in claims data, healthcare organizations can implement proactive measures to prevent fraud, ultimately reducing financial losses.
Telemedicine, telehealth triage
Predictive algorithms work here to triage patients in telehealth settings, determining the urgency of care based on symptoms and medical history, and predict health deteriorations remotely by monitoring patient data, enabling timely interventions and reducing hospital admissions.
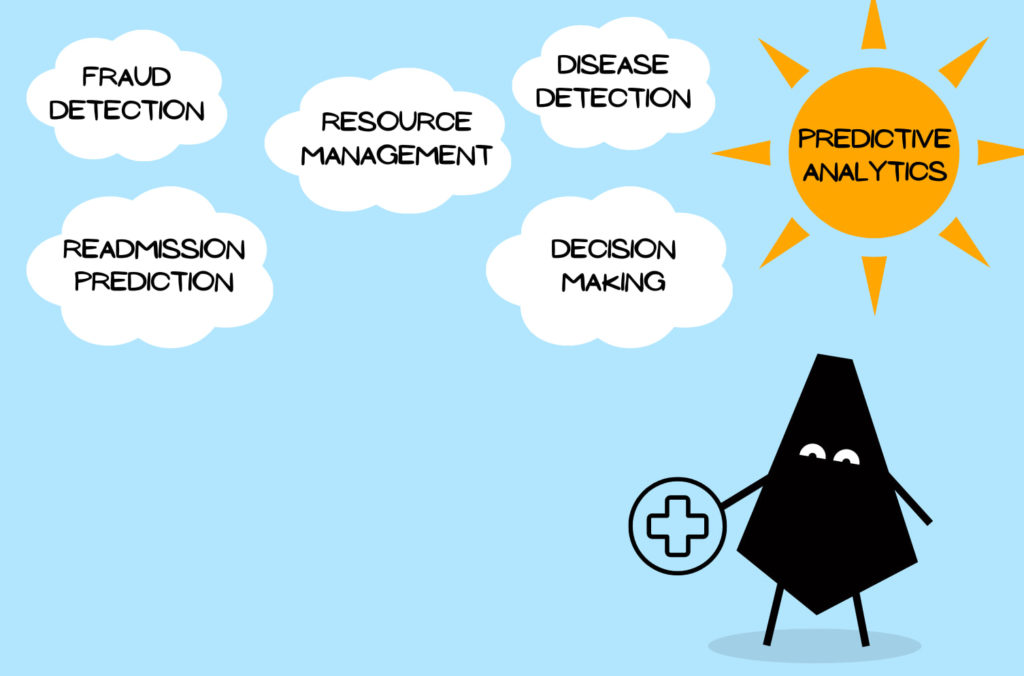
What means do you need for efficient usage of predictive analytics in healthcare?
To get the most out of predictive analytics, you are supposed to use appropriate technologies, tools, and platforms. To that end, you may consider the following:
AI role for predictive analytics in healthcare
AI is the number one technology in predictive analytics in healthcare, bringing advanced capabilities to analyze vast and complex datasets, extract meaningful patterns, and make accurate predictions about patient outcomes and health-related events. Moreover, AI combined with IoT can also create miracles in healthcare.
- Advanced algorithms for predictive modeling: AI encompasses a range of advanced algorithms within the field of machine learning, such as decision trees, random forests, support vector machines, and neural networks. These algorithms can analyze historical data to make predictions about future health events, patient outcomes, or disease occurrences.
- Natural Language Processing (NLP): NLP enables the extraction of valuable insights from unstructured text data, such as clinical notes, medical literature, and patient narratives. By understanding and processing natural language, AI helps incorporate textual information into predictive models, enriching the analysis.
- Deep learning for complex patterns: Deep learning, a subset of machine learning, involves neural networks with multiple layers capable of learning intricate patterns and representations. In healthcare, deep learning is applied to tasks such as medical imaging analysis, diagnostics, and predicting disease progression.
Patient journey mapping
Patient journey mapping is another essential tool in predictive analytics for healthcare. It involves tracking and analyzing the entire patient experience, from the first point of contact to post-treatment follow-ups. By mapping the patient journey, healthcare providers can identify critical touchpoints, potential bottlenecks, and areas for improvement. This comprehensive view enables the prediction of patient needs, preferences, and potential issues, facilitating a more personalized and effective healthcare experience.
Predictive modeling in healthcare
One of the key techniques employed in predictive analytics is predictive modeling. This involves the creation of a mathematical model based on historical data to predict future events or outcomes. In healthcare, predictive modeling can be applied to various aspects such as patient admissions, disease progression, and treatment efficacy. Understanding and implementing effective predictive modeling techniques is crucial for healthcare providers to harness the full potential of predictive analytics.
Tableau predictive analytics
Tableau, a popular data visualization tool, plays a vital role in predictive analytics in healthcare. Its capabilities extend beyond just displaying data. Tableau can integrate predictive analytics models seamlessly. Healthcare professionals can use Tableau to create interactive and visually compelling dashboards that incorporate predictive insights. This not only facilitates better decision-making but also enhances communication and collaboration among healthcare teams. Its key features are:
- interactive and user-friendly dashboards;
- integration with various data sources;
- visualization of predictive model outputs.
Cloud-based predictive analytics platforms
- IBM Watson Analytics: it is a cloud-based analytics platform that leverages AI and machine learning for predictive analytics. Its key features include (i) natural language processing for easier interaction, (ii) automated predictive modeling, and (iii) integration with IBM’s other healthcare solutions.
- Microsoft Azure Machine Learning: Azure ML is a cloud-based platform by Microsoft that provides tools for building, deploying, and managing machine learning models. Its key features include (i) seamless integration with other Azure services, (ii) a drag-and-drop interface for model development, and (iii) scalability and flexibility for large datasets.
- Google Cloud AI Platform: it offers a suite of tools and services for building and deploying machine learning models on Google Cloud. Its key features include (i) pre-built machine learning models, (ii) integration with other Google Cloud services, and (iii) scalable infrastructure for processing large datasets.
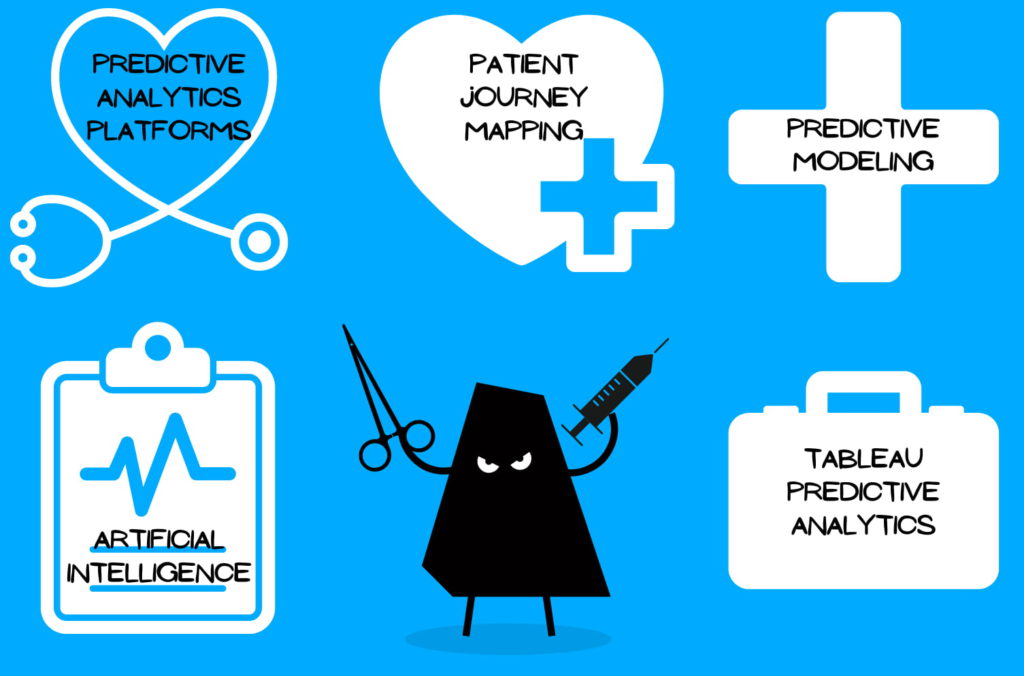
What else to consider in predictive analytics in healthcare?
Data quality in healthcare
One of the foundational aspects of effective predictive analytics in healthcare is data quality. Reliable and accurate data are essential for building robust predictive models. By ensuring the integrity of healthcare data, providers can trust the predictions generated by analytics tools. Strategies for maintaining data quality, including regular audits, standardization, and data cleansing, are crucial for the success of predictive analytics initiatives.
Data integration and interoperability
Ensuring seamless integration of data from various sources and systems is important. Interoperability allows for a holistic view of patient information, improving the accuracy and completeness of predictive models. To be integrated properly, electronic health records (EHRs), claims data, patient demographics, and other relevant information should be accurate, up-to-date, and readily available.
Data governance and standardization
Robust data governance practices shall be established to maintain data quality, consistency, and security. Furthermore, it is required to standardize data formats and terminologies to enhance interoperability and facilitate analysis.
What can you gain from predictive analytics in healthcare?
Data-driven healthcare
Predictive analytics transforms healthcare into a data-driven industry. The abundance of health data, including electronic health records, wearable device data, and genetic information, is harnessed to derive actionable insights. This data-driven approach allows healthcare providers to make informed decisions, optimize resource allocation, and improve patient outcomes. The integration of predictive analytics into daily workflows empowers healthcare professionals with timely and relevant information.
Conclusion: making patient journey unforgettable with predictive analytics
To summarize, the integration of predictive analytics in healthcare holds a significant place as it enables healthcare providers to move beyond traditional reactive methods to a more anticipatory and preventive healthcare model. By embracing predictive modeling, patient journey mapping, and leveraging tools like Tableau, healthcare providers can unlock new dimensions of patient care.
The healthcare sector has witnessed a notable shift from the traditional fee-for-service model to a value-based care approach. Predictive analytics aligns seamlessly with this shift, as it allows healthcare providers to optimize resources, improve patient outcomes, and enhance overall efficiency. The ability to anticipate, mitigate, and personalize care not only improves outcomes but also shapes a future where healthcare is not just about treating illnesses but about fostering enduring patient-provider relationships through predictive insights.