Big Data and Pharma: Building Preclinical and Clinical Analytics Platforms
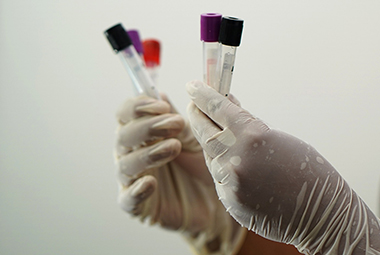
Living through a pandemic in the past hectic year, healthcare professionals and providers work even harder in order to provide us with relevant research insights and medicines. These external circumstances gave a significant push for further and more intense digitalization, so the role of technology in the pharma sector has never been more critical.
Just recently, a group of MIT scientists have announced a new Machine Learning approach to finding drugs effective against COVID-19 from those that are already on the market. Virtual screening has been used by scientists in China for a similar purpose, finding an existing lymphoma drug for virus treatment. Though media highlights are shifted mainly to those dealing with COVID, researchers working on other treatments are successful in implementing current tech and analytics in pharma research. AI and ML, bioprocessing and other analytical devices have uncovered numerous new targets and medicines in the past year.
To keep up with the changing pharmaceutical industry and its challenges, it is essential to utilize technologies based on data and data analytics. Data analytics platforms enable these solutions for health companies and organizations. In continuation of our previous article on how data analytics can improve the pharma industry, we will look into architecture of analytics platforms for pharma, specifically preclinical and clinical software.
Building Preclinical and Clinical Solutions: Goals and Needs
Due to the sensitive and precise information they work with, preclinical and clinical development operations require applications of high scientific standards. Drug development and trials are not only regulated by HIPPA, but also by FDA’s GLP, ICH Safety Guidelines, Control of Substances Hazardous to Health guideline, various animal testing and human drug trial regulations. Not to mention rigorous security, low error bar, accurate calculations, well-structured models needed to ensure productive research results. Varying among the specific tasks and needs a company, organization or laboratory has, the most used types of clinical and preclinical software are responsible for:
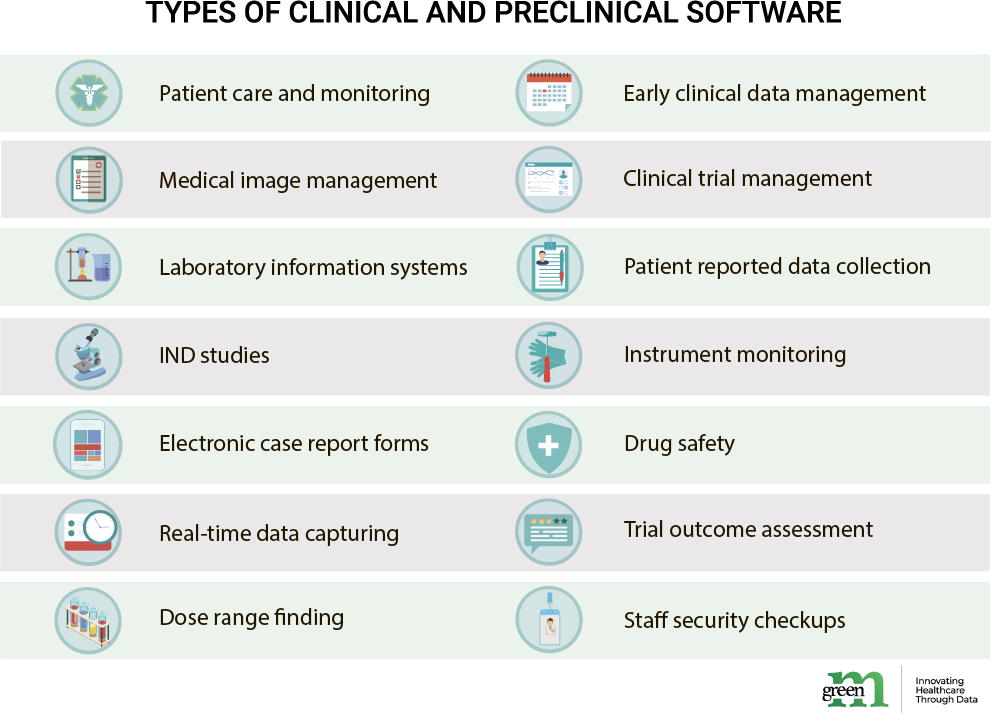
Though there might be separate solutions for each stage of drug development and testing, having numerous distinct applications may not always be a good idea. Why? Due to the distinct data formats pharma works with, it is likely that either data, metadata, claim forms or insights will be unreadable from platform to platform, which may result in a need for tweaking the software or re-coding the data itself. This takes additional time by increasing the number steps in the research process. Additionally, the transfer of data cross-platform may open it to security compromises. Among other challenges facing when digitalizing are access to data cross-application (which may be problematic if not impossible all together), data heterogenous source or its uneven flow.
As a reasonable alternative, many pharmaceutical companies choose investing into comprehensive applications. Data analytics platforms are among the most common examples of such software. They are ecosystems of services and technologies, which analyze big data in order to provide BI, medical predictions and visualizations for further decision-making. Complex research tasks such as therapeutic antibody screenings or RNA mapping can be automated and enhanced by these technologies. From the business side, data analytics platforms can analyze and predict drug demand, automate QA process, even provide built-in CRM solutions.
Top 3 Prerequisites for Implementing Data Analytics in Pharma
Since the preclinical and clinical pharmaceutical development is a very distinct field, it has its own requirements to facilitate successful analytics. Firstly, understanding of the pharmaceutical industry at large, its trends is a certain prerequisite for a company in order to develop a resilient analytics platform.
Secondly, knowledge of business needs of a specific company is a must too. Since data analytics are built to answer specific business and research problems, niche and context matter. Large scale multi-trial research with thousands of patients will need a platform focused on user accessibility and scalability, while a company doing bioengineering and chemistry may need more computing, ML and raw data processing.
Though varying in its applications, the core of analytics is always data. Therefore, the third prerequisite is available digitalized datasets for further processing. Often these are vast data lakes of code research and testing data, organizational data or patient reports, among others. What makes pharma field special are mixes of structured information and unstructured media, variety of standards, protocols, and formats.
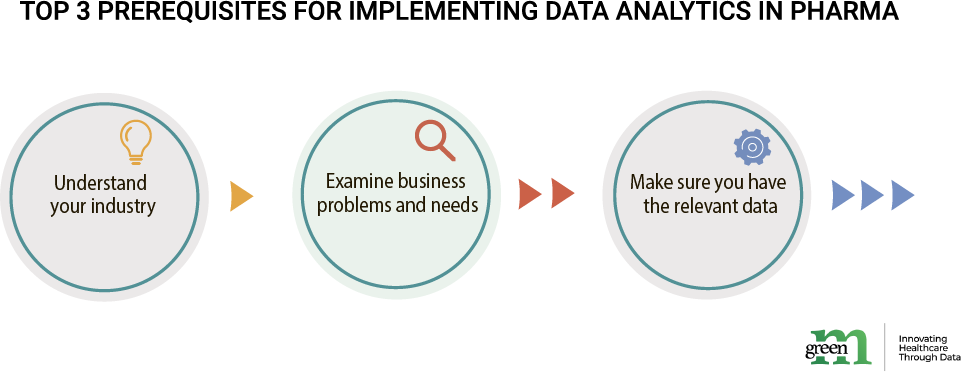
Design of a Pharma Data Analytics Platform
To implement the best solutions for pharma, developers and business professionals collaborate to create architectures that combine a set of tools and technologies pharmaceutical organizations use to manage data and address their goals. Prioritizing use cases for your data is essential for a large project like this in order to maximize its impact and value. So is careful planning. Therefore, a clear goal-setting and thorough risk assessment are the key to-dos before designing an analytics platform or improving an existing one. A few key questions to consider are:
- What organizational questions need to be answered?
- Which KPIs do you want to measure?
- Who are your users and stakeholders?
- What data is available?
- What are your security needs?
- Can you automate?
- Are you planning to scale?
- How will you do integration?
- What are the data types you work with?
- Which technology stack do you want to use?
- What are the potential foreseeable issues to consider?
Simplified, analytic platform architecture consists of the three large tiers that are tightly interconnected: data and statistics, middleware and analytics, reporting and visualization.
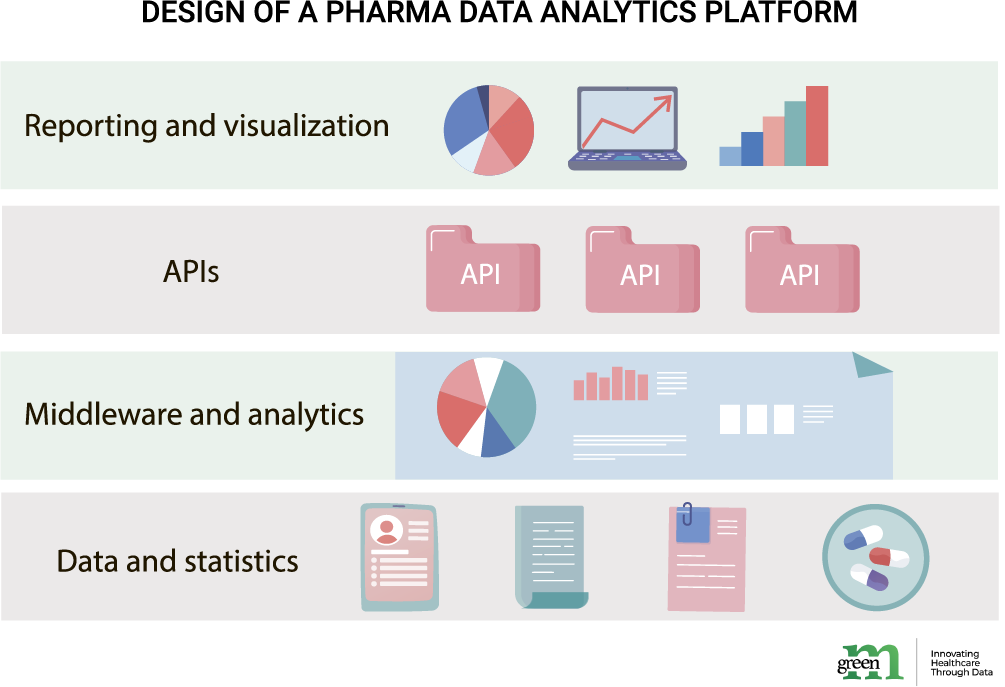
Data and statistics tier
This layer is the whole repository of the data available to you. Within the specificity of preclinical and clinical software, these may be clinical data and metadata, information about drugs and ingredients, patient information or reports. Additionally, this tier is responsible for data quality profiling and its cleaning and preparation for the analytics process that comes next.
Middleware and analytics tier
The second process is the essential analytics of the said data repository. Consolidated data is passed towards message-oriented middleware (MOM) and APIs, allowing for data units to interact or be compared. Models to use for the analysis itself may vary significantly based on a specific company’s priorities and KPIs. Correlation and dependence models, probability distribution measurements, virtual screenings, text and image analytics, pattern matching, cross-source information comparison, ML and AI – all are viable analytical algorithms for successful result-generation.
PARTNER UP WITH DATA EXPERTS
We help to deliver pharma data solutions to address questions and issues relevant to your company’s needs. Build a versatile data platform, solve data challenges and speed up delivery.
Reporting and visualization
The aim of a pharma analytics platform is to provide actionable insights. Third tier is responsible exactly for that. Report generation are useful for researchers in further development of their treatments. Data-backed resource planning, workflow management and on-demand decisioning are game changers in corporate and business decisions. In cases where complex medical data is to be presented for business associates, science journals or patients, readability and user-friendliness of data reports may be a requirement. In this case, visualization of insights can often help with that task, while automating it completely takes a large lump of work off researchers’ shoulders.
Key Takeaways
As any healthcare IT solution, building preclinical and clinical analytic platforms requires not only a secure top-tier application structure, but also an in-and-out understanding of the healthcare business and research specificities.
It is important to adhere to HIPPA, GLP and ICH Safety Guidelines when building an IT solution for research.
Obtaining a relevant organizational, testing and patient data is fundamental for insightful analytics.
Pharma analytics platform architecture consists of three large tiers: data and statistics tier (data pool), middleware and analytics tier (MOM, APIs and analytics models), reporting and visualization (insights, tables, charts and reports).
While it is easy to get lost in the endless solutions, prioritize analytics models suitable for your specific needs.
Want to stay in the loop? Subscribe to GreenM Health Tech Digest and get top 5 handpicked industry insights, cases and business recommendations in your inbox every two weeks. Hit the SUBSCRIBE button below to learn about digital health with our newsletter or read more news here!
WANT TO BUILD A HEALTHCARE MVP BASED ON OPEN DATA?
Learn how to take a concept from a business problem to a functioning solution in a very short period.
References
1. McKinsey COVID-19 Consumer Survey https://www.mckinsey.com/
2. A machine-learning approach to finding treatment options for Covid-19 | MIT News | Massachusetts Institute of Technology https://news.mit.edu/2021/machine-learning-treatment-covid-19-0216
3. New virtual screening strategy identifies existing drug that inhibits COVID-19 virus (worldpharmanews.com) https://www.worldpharmanews.com/research/5523-new-virtual-screening-strategy-identifies-existing-drug-that-inhibits-covid-19-virus
4. 2019 Pharma Innovation Awards (pharmamanufacturing.com) https://www.pharmamanufacturing.com/articles/2019/2019-pharma-innovation-awards/
5. Exonate launches diabetic macular oedema trial – PharmaTimes
http://www.pharmatimes.com/news/exonate_launches_diabetic_macular_oedema_trial_1362699
6. Pre-Clinical Testing – Regulatory Roadmap (therqa.com) https://www.therqa.com/good-practices/regulatory-roadmap/pre-clinical-testing/
7. Regulations: Good Clinical Practice and Clinical Trials | FDA https://www.fda.gov/science-research/clinical-trials-and-human-subject-protection/regulations-good-clinical-practice-and-clinical-trials
8. Clinical Trial Regulation | European Medicines Agency (europa.eu) https://www.ema.europa.eu/en/human-regulatory/research-development/clinical-trials/clinical-trial-regulation
9. Big Pharma – Drug & Device Companies, Lawsuits & Facts (drugwatch.com) https://www.drugwatch.com/manufacturers/
10. Understanding Pharmacy Management Tech Solutions (drugtopics.com) https://www.drugtopics.com/view/understanding-pharmacy-management-tech-solutions
11. Build Data and Analytics Leadership Traits for Digital Business (gartner.com) https://www.gartner.com/en/information-technology/role/it-data-analytics-leaders
12. Building a Successful Modern Data Analytics Platform in the Cloud | by ML-Guy | Medium https://guyernest.medium.com/building-a-successful-modern-data-analytics-platform-in-the-cloud-4be1946b9cf5
13. How pharma companies are applying advanced analytics to real-world evidence generation | McKinsey https://www.mckinsey.com/industries/pharmaceuticals-and-medical-products/our-insights/creating-value-from-next-generation-real-world-evidence